
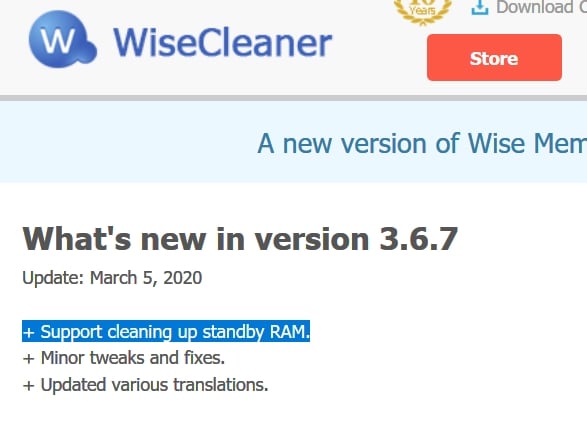
Secondly, different variants of attention mechanisms are applied to enhance the interaction between fine-grained visual features and language features. Firstly, on data representations, global features are replaced by image regions features and words features, which enable the model to fuse modal information at a finer level.

To address the problem, two lines of work have made major contributions and shown effective improvement in accuracy. Inchoate methods coarsely learn joint embedding representation with global features, which contains more noise and has difficulty answering the fine-grained questions.
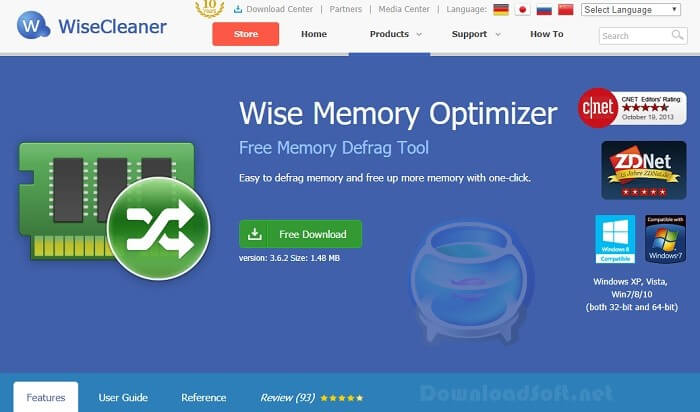
The proposed PAM is beneficial to construct a robust multi-hop neighborhood relationship between visual and language and achieves excellent performance on both VQA2.0 and VQA-CP V2 datasets. Moreover, we use guard gates of the target modal to check the source modal values in CA and conditioning gates of another modal to guide the query and key of the current modal in SA. Four memoried single-hop attention matrices are used to obtain the path-wise co-attention matrix of path-wise attention (PA) therefore, the PA block is capable of synthesizing and strengthening the learning effect on the whole path. After each single-hop attention block (SA or CA), the importance of the cumulative nodes is used to calibrate the signal strength of nodes’ features. We propose a path attention memory network (PAM) to construct a more robust composite attention model. However, the existing composite models only consider the stack of single attention blocks, lack of path-wise historical memory, and overall adjustments. One main solution is the composite attention model which is composed of co-attention (CA) and self-attention (SA). Visual question answering (VQA) is regarded as a multi-modal fine-grained feature fusion task, which requires the construction of multi-level and omnidirectional relations between nodes.
